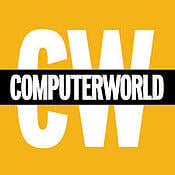
There's no wizardry, no crystal ball. The retailer relies on the science of predictive analytics, using technologies from IBM to forecast demand for its line, which it sells through Nordstrom and other high-end retail stores. The tools pull data from a continuously updated data warehouse to forecast what needs to ship to each store every week, right down to the styles, colors and sizes each location will need to meet demand.
"That protects the customer, ensuring that any style or color they order is in stock, but also protects us so we don't overproduce," says Nihad Aytaman, director of business applications at Elie Tahari.
Analytics have made an indelible mark on the retail fashion business over the past decade, helping with everything from predicting the best pricing and markdown strategies to forecasting the right mix of products, colors and sizes for every location. There's one critical area, though, that Elie Tahari and many other retailers and designers still don't use predictive analytics for: choosing which new styles will be next season's winners.
But thanks to new technologies, that could be changing.
"Maybe tie-dye is going to be huge or pink will be big. Those are decisions that the merchant has always made, but that can be assisted with sophisticated algorithms that point out patterns that [they] may have missed," says Cathy Hotka, principal of retail consulting firm Cathy Hotka & Associates.
Predictive analytic tools, which rely on historical data to make future demand projections for any given product, can play a role even in predicting the whims of fashion. But right now, the hottest area for picking fashion winners lies at the intersection of analytics and social media.
While predictive analytics can help identify fashion winners, most merchandisers aren't using the technology for that purpose, for two reasons: Unlike products that are carryovers or that will simply be revised for the next season, new fashions don't have the historical sales data that predictive analytic tools need to work their magic, and retail buyers are wary of allowing science to intrude on the art of picking fashion winners.
"For us right now, key styles are picked by merchants in their discussions with designers, who present products that are inspired by trends and what's happening in the world," says Louise Callagy, a spokesperson for Gap Inc. But Gap expects analytics to play a bigger role in the future. "Although it's in the early stages, we apply analytics from our early online sales globally and in certain markets to help gauge a better read of what we predict will sell in stores," she says.
High-stakes Game
"Computer-aided fashion projections are something everyone is talking about," says David Wolfe, creative director at The Doneger Group, which predicts fashion trends the old-fashioned way: using seasoned experience and insight. But it's a high-stakes decision for merchandisers and fashion designers -- and one that can be tricky to get right. Fashion retailers stake their fortunes on the experience, intuition and gut instincts of an elite cadre of buyers. For smaller retailers, the effect of a buyer who loses his mojo can be devastating to the bottom line.
"Apparel is a very fickle business. If you miss one season, you can go under," says Aytaman. Most buyers simply don't trust technology to do the job. So they turn to consultants like The Doneger Group for predictions as to what colors and styles will be in -- and what will be out. Those insights, in turn, are based on experience, intuition and regular visits to designers and fashion shows.
Adding to the pressure is the fact that the consumer market has fragmented and shoppers are less willing to embrace styles dictated from the runway or by designers and retailers. Just 19% of consumers listen to manufacturers or retailers these days, according to an IBM survey. Consumers today tend to make their own decisions about fashion, in conjunction with their peers. More than ever, the industry needs to listen to the customer.
The Elements of Style
The problem with using predictive analytics to forecast fashion trends, says Aytaman, is that the accuracy of those predictions varies in direct proportion to the amount of historical data that can be fed into the model. So while Elie Tahari uses analytics to determine, for example, demand for its business-suit line, which doesn't change much from year to year, it doesn't use the technology to pick more seasonal, fashion-oriented items, such as dresses and sportswear.
"We can't accumulate enough history to really do something like this," he says.
While it's true that a new design may have no historical analog on which to model success, merchandisers can break down the key attributes that describe a given fashion -- everything from color to collar size -- and perform a regression analysis on those. In other words, merchandisers can perform a statistical analysis on all of the variables that describe the new style, assuming historical data is available, to project whether the item will be hot or not.
"Using attributes and supplementing that with what you see as fashion trends, again as attributes, is pretty cutting edge," says Saurabh Gupta, a senior solutions specialist at SAS, who is responsible for product management of retail solutions. And while there may not be enough historical data to create models for every attribute, he says some fashion elements do have predictable cycles. "A color stays popular for a year at least, and you can derive insight from that," Gupta says. (Editor's note: An earlier version of this story incorrectly identified Gupta as a director at IBM.)
And retailers can enhance models with knowledge, such as the fact that certain types of fabrics are becoming less attractive to buyers. "It's about bringing in extra evidence, not one killer attribute," says Colin Linsky, predictive analytics worldwide retail sector leader at IBM. But the real value of predictive analytics in fashion is not just that it can pick winners, Linsky says. "It also gives a strong indication of the why, and that's important in understanding what you should be doing when making merchandising decisions," he says.
On the other hand, predictive analytics doesn't always work as well when a new fashion doesn't follow previous patterns, when there's limited or no historical data for key attributes, or when the style falls into a different line, such as when it moves from dresses to sweaters, says the CIO of a large fashion designer and retailer that sells online and through more than 500 stores, who spoke on the condition that his name and company (we'll call it Company Z) not be identified.
"Someone has to model that based on their knowledge, and that's where the art of merchandisers comes into play," the CIO says. "You still hear in the buying meetings, we believe this will happen. This is the forever battle of science versus art."
But none of this will work, he says, unless the right systems are in place to supply the same data, consistently, to all parts of the business. At Company Z, that means having a master data model and an enterprise service bus to move the data between subsystems, and to share data across sales channels and buyer silos. And final validation requires human review and approval across all functional areas, including plan allocation, production sourcing and finance, as well as approval by the merchants.
"At the end of the day, if you don't have good data you use across the enterprise, the results aren't the same," the CIO says. "That's very important to predictive systems."
The CIO's company isn't the only retailer doing this, but it's ahead of the curve, according to IBM's Gupta. "Everyone says they understand attributes, but how to use them to predict demand is not something a lot of companies do well."
Mining Social Intelligence
To augment traditional analytics, some retailers and fashion designers have applied analytic techniques to social media interactions to get real-time feedback on where fashion is going and what consumers think of their upcoming designs.
Social analytics are changing the game in retail, says Doug Stephens, president of research consultancy Retail Prophet. "We're moving from an outside-in approach, to a world where inventory and demand planning and product development will all be driven by social media," he says.
At one large retailer that creates its own fashions, designers use the feedback in an iterative loop to evolve fashion items, tuning each for the most enthusiastic consumer response, according to an IT executive who spoke anonymously.
First Insight offers a service that tests how consumers will react to new fashions by engaging them in activities, such as playing games at social media sites. "The application can be used for high-fashion items where there is very little history," says Greg Petro, the company's CEO. First Insight asks users what they think others would pay for test products and gauges their general sentiment about them.
What makes the results different from a focus group is that First Insight determines the "predictive relevancy" of participants' responses by seeding the exercise with products with known outcomes. It examines how their predictions match up with what actually happened with those items, assigns a weighted predictive value to each user, and factors that in when aggregating the results to predict winners and losers for the fashions on which they're building a demand prediction.
Deliverables include not just which products will sell, but suggested price ranges as well. The application is particularly useful for predicting consumer response to high-fashion items that have little or no history to go on, says Petro.
Wild Things LLC, a manufacturer of military and alpine clothing and related gear, was one of First Insight's first customers. CEO Ed Schmults, who is now on the vendor's advisory board, says he first used the service to choose the best style for a corporate logo and is using it to gauge consumer reactions to clothing styles that will launch next year under its newly licensed Smith & Wesson brand.
"Our consumer lines are absolutely driven by fashion. We want to understand customer receptivity to the product, the color, the price point," he says. "This is a very powerful tool for moderating that risk."
Elie Tahari looked at First Insight's technology, and while Aytaman says it was technically "pretty accurate," it went nowhere with store buyers. "Although they liked the idea, they didn't trust it," he says.
Gilt Groupe, which offers members-only flash sales of high-fashion items online, uses a combination of traditional analytic tools from SAS and collective intelligence from a startup company to predict which styles or brands will be winners. Stylitics, a social networking site launched this summer, uses a methodology similar to that of First Insight, but it focuses on the consumer's intentions and what they already have purchased rather than on how they think others would react to a fashion or product line, says Tamara Gruzbarg, senior director of analytics and research at Gilt (see sidebar, below).
Four years ago, Gilt knew exactly what its customers' tastes and brand preferences were. Today, customers are less brand-oriented, so Gilt relies on predictive analytics to help buyers understand what will sell. But, Gruzbarg cautions, you have to know what you're looking for. "The analytic tools are only as good as the data on which you're elaborating. Understanding what the most relevant information is, that's critical," she says.
Manya Mayes, director of predictive analytics at Attensity, says text analytics are being used on data provided from social media sites such as Storify, which lets online users create their own visual stories about what outfits they like. "The analytics identify which clothing combinations are put together most often and which ones they are keeping," she says.
Merchants are also mining "fashion haul" videos, in which teens show off goods they bought at the mall and voice strong opinions about them. Some fashion haul posts have gone viral, with as many as 1 million hits in the first week, says Jill Puleri, vice president of global retail at IBM, citing videos by young women named Blair Fowler, Ellie and Fiona. "That's something you can input into your trending models," she says.
Predictive analytics reduces the overall risk on fashion selections, allowing the business to take some chances, says Schmults. "The art is introducing things that consumers wouldn't have thought about before," he says.
Crowdcast offers a different spin on collective intelligence. Its service lets employees within an organization, such as buyers, store managers or employees, bet virtual money on which products will be winners.
"The collective wisdom of several merchants is usually better than the single estimation of one," says Greg Girard, an analyst at IDC. In the Crowdcast model, participants win more money when they're right, allowing them to place bigger bets, which gives them greater weight when all bets are tallied. In this way, he says, a group of buyers can all bet on this season's line of clothing.
So far, most users have been manufacturers, which use the tool to predict when products will ship or how well they will sell, but Crowdcast is pitching it to fashion retailers. "When you have very little data to make big decisions, that's where you can benefit from collective intelligence expertise," says Mat Fogarty, the company's founder and CEO.
Timing is another challenge. It's not enough to know that a fashion item keys into a popular trend, says Company Z's CIO. Retailers need to know when those trends will hit. Company Z uses crowd-sourcing and collective intelligence tools similar to what First Insight and Crowdcast offer. But it also does test marketing in stores and through its e-commerce channel and then feeds the results into its data warehouse, where it's used as additional input for its predictive modeling engine.
"Predictive analytics doesn't change the way we run our business," the CIO says. "All it does is streamline the processes so we're more analytical."
Pulling It Together
The impressions and insights from social media analytics can be fed into traditional predictive analytic engine models, providing another input to help determine fashion winners, says IBM's Linsky. First Insight's data can fit within predictive analytic data models, says Petro. "It's just a matter of mapping it," he explains.
Going forward, social analytics will reshape the merchandiser's job into "social merchants," says Girard. But for now, using analytics -- social or otherwise -- to pick fashion winners is still a "missionary market," with many retailers still on the sidelines, merchants and designers not completely sold on the idea, and everyone waiting for the first big success story.
As for cultural resistance, Petro thinks the technology will gradually win over merchants as they see the results and understand where the tools fit. Predictive analytics is no substitute for human judgment, he says: "It's an instrument in the cockpit, not a replacement for the pilots themselves."
Read full article: http://www.computerworld.com/s/article/357932/The_Art_Science_of_Fashion?pageNumber=4